Software predicts future cell health
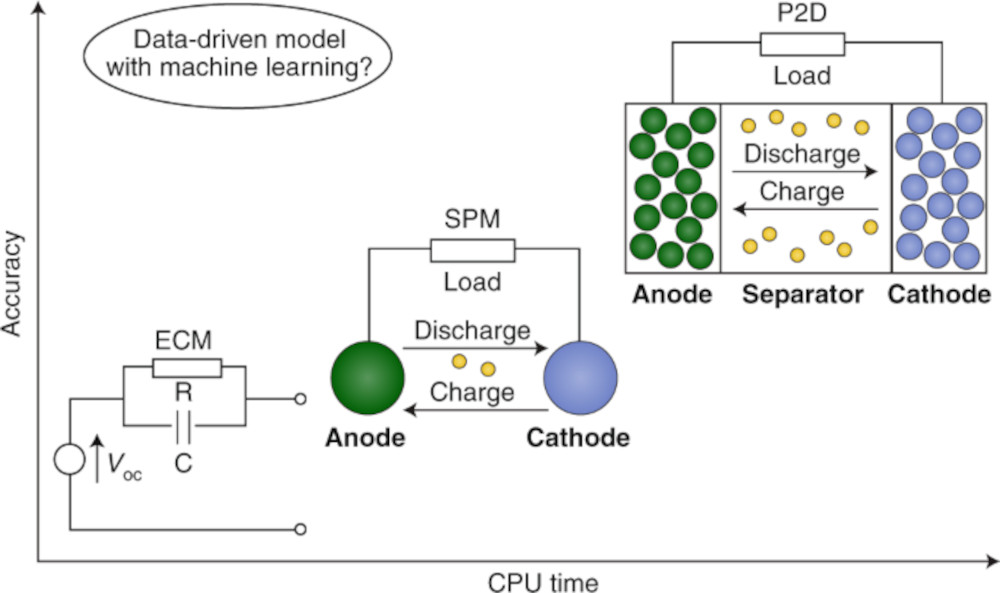
A machine learning algorithm could help reduce charging times and prolong battery life in EVs by predicting how different driving patterns affect battery performance (writes Nick Flaherty).
Researchers from the University of Cambridge in the UK developed a non-invasive impedance-based approach to get accurate data on the state of health (SOH) of a battery. The results were then fed into a machine learning algorithm that can predict how different driving patterns will affect the future health of the battery.
The algorithm could be used to recommend routes that get drivers from point to point in the shortest time without degrading the battery, for example, or recommend the fastest way to charge the battery without causing it to degrade.
“Most methods of monitoring battery health assume that a battery is always used in the same way, but that’s not how we use batteries in real life – how you drive will affect how the battery degrades,” said Penelope Jones, a researcher from Cambridge’s Cavendish Laboratory, who worked on the project.
The problem is that batteries with the same numerical SOH do not necessarily exhibit identical levels of each degradation process – lithium plating or electrode cracking, for example – yet the impact of future cell usage on the cell’s performance and degradation pathway depends significantly on the type of degradation that has already occurred.
A monitoring system is therefore needed. The researchers’ non-invasive monitoring probe sends electrical pulses into a battery and measures the response, providing a series of ‘biomarkers’ of battery health. The method is gentle on the battery and doesn’t cause it to degrade any further.
The electrical signals from the battery were converted into a description of the battery’s state, which was fed into a machine learning algorithm. The algorithm was able to predict how the battery would respond in the next charge-discharge cycle, depending on how quickly the battery was charged and how fast the car would be going the next time it was on the road.
Tests with 88 commercial batteries showed that the algorithm did not require any information about previous usage of the battery to make an accurate prediction.
The experiment focused on lithium cobalt oxide cells, which are widely used in rechargeable batteries, but the method is applicable across the different types of battery chemistries used in EVs.
The method can be used by manufacturers, end-users or recyclers because it allows the health of the battery to be captured beyond a single number, and because it’s predictive. It could also reduce the time it takes to develop new types of batteries, allowing researchers to predict how they will degrade under different operating conditions.
The researchers are now working with battery manufacturers on next-generation batteries and how the framework could be used to develop optimal fast-charging protocols to reduce EV charging times without causing degradation.
ONLINE PARTNERS
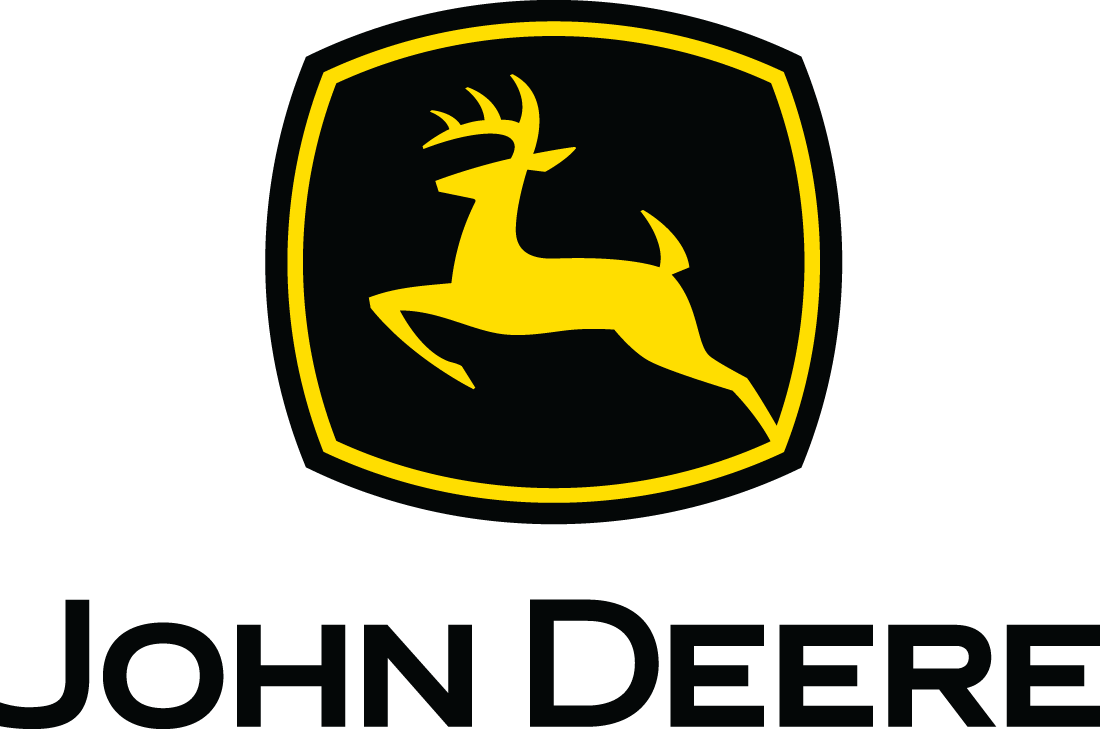
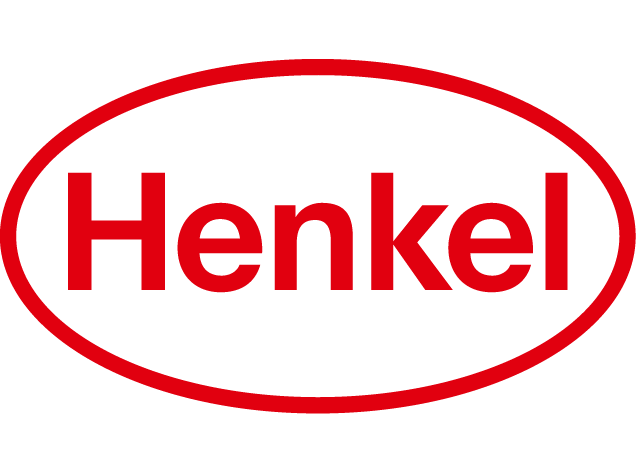
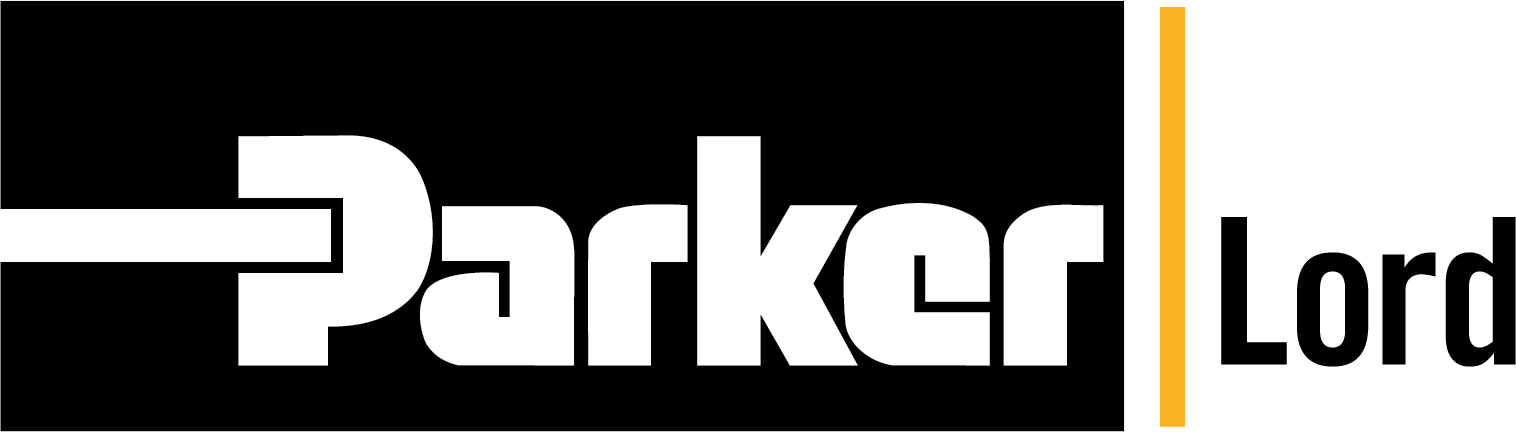
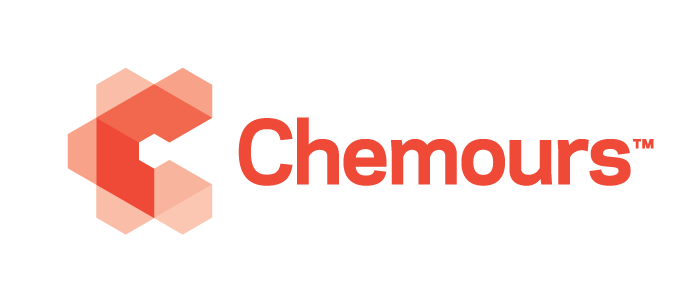
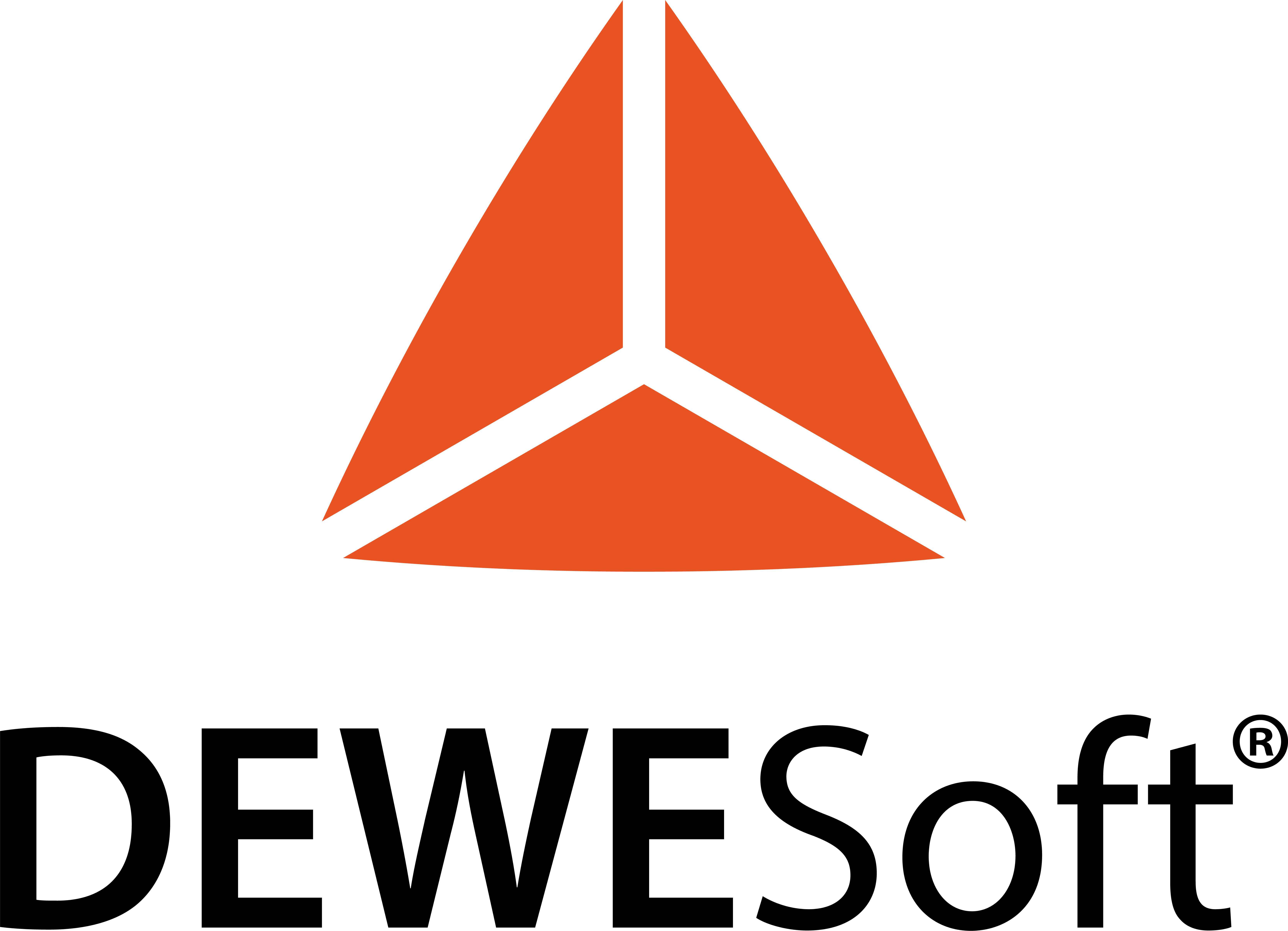
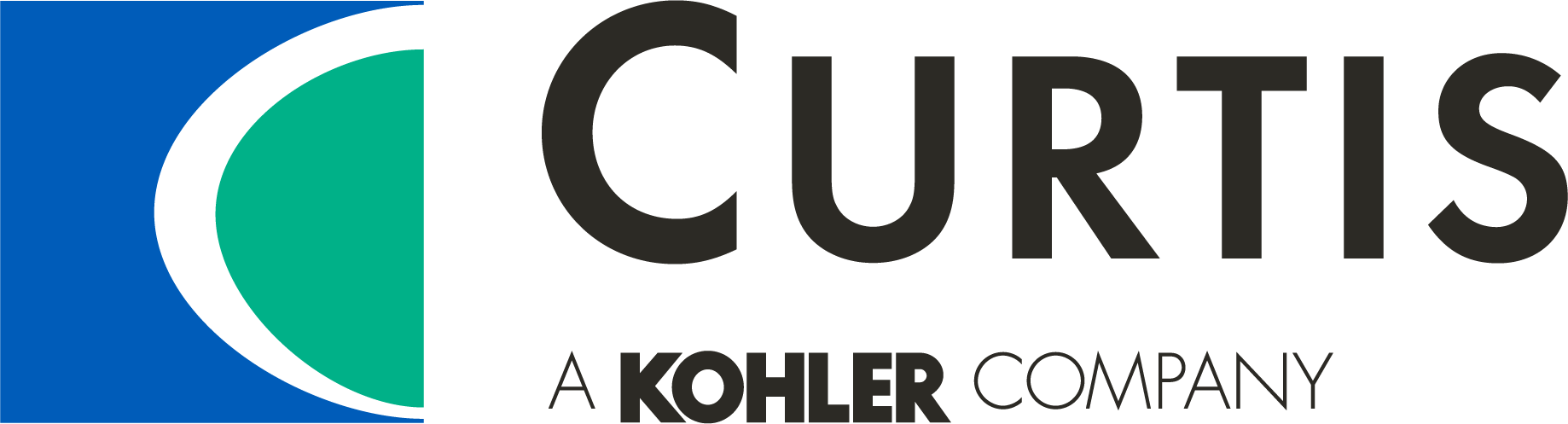
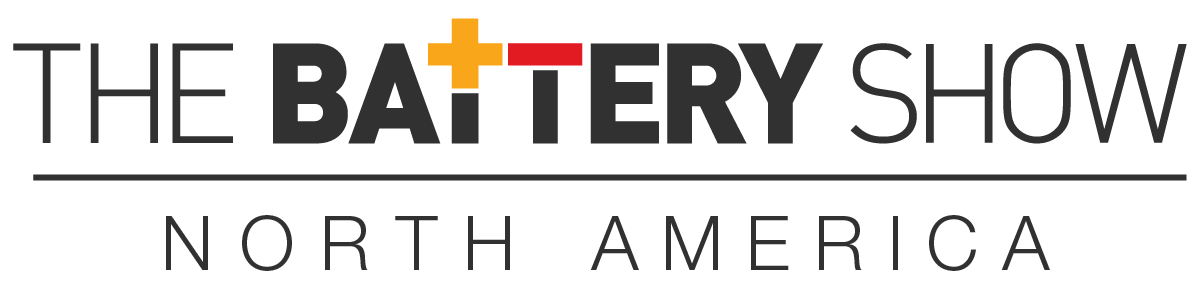
