ML finds solid-state cell materials
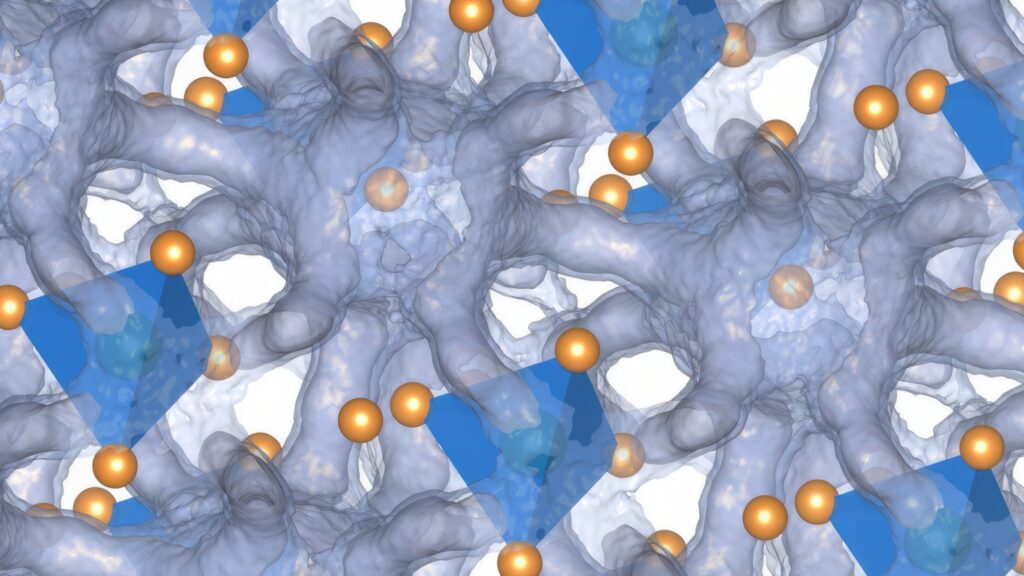
Researchers in the US have used machine learning (ML) to identify new combinations of materials for solid-state batteries (writes Nick Flaherty).
The class of compounds, called argyrodites, contains attractive candidates for solid-state battery electrolytes. They are built from specific, stable crystalline frameworks made up of two elements, with a third free to move about the chemical structure. While some recipes such as silver, germanium and sulphur are naturally occurring, the general framework is flexible enough for researchers to create an array of combinations.
“Every EV manufacturer is trying to move to new solid-state battery designs, but none of them are disclosing which compositions they’re betting on,” said Olivier Delaire, associate professor of mechanical engineering and materials science at Duke University.
Delaire and his colleagues looked at one promising candidate, made of silver, tin and selenium. Using a combination of neutron beams and X-rays, the team captured data to use in an ML approach to build a computational model to match the observations using first-principles quantum mechanical simulations.
The results showed that while the tin and selenium atoms created a relatively stable scaffolding, it was far from static. The crystalline structure constantly flexes to create windows and channels for the charged silver ions to move freely through the material. The tin and selenium lattice remains solid, while the silver is in an almost liquid-like state.
Combining advanced experimental spectroscopy with ML will help researchers develop new materials for solid-state batteries using different recipes. One combination that replaces the silver with lithium is of particular interest to the group, given its potential for EV batteries.
“Many of these materials offer very fast conduction for batteries while being good heat insulators for thermoelectric converters, so we’re systematically looking at the entire family of compounds,” Delaire said.
“This study serves to benchmark our ML approach that has enabled tremendous advances in our ability to simulate these materials in only a couple of years. I believe this will allow us to quickly simulate new compounds virtually to find the best recipes of them.”
ONLINE PARTNERS
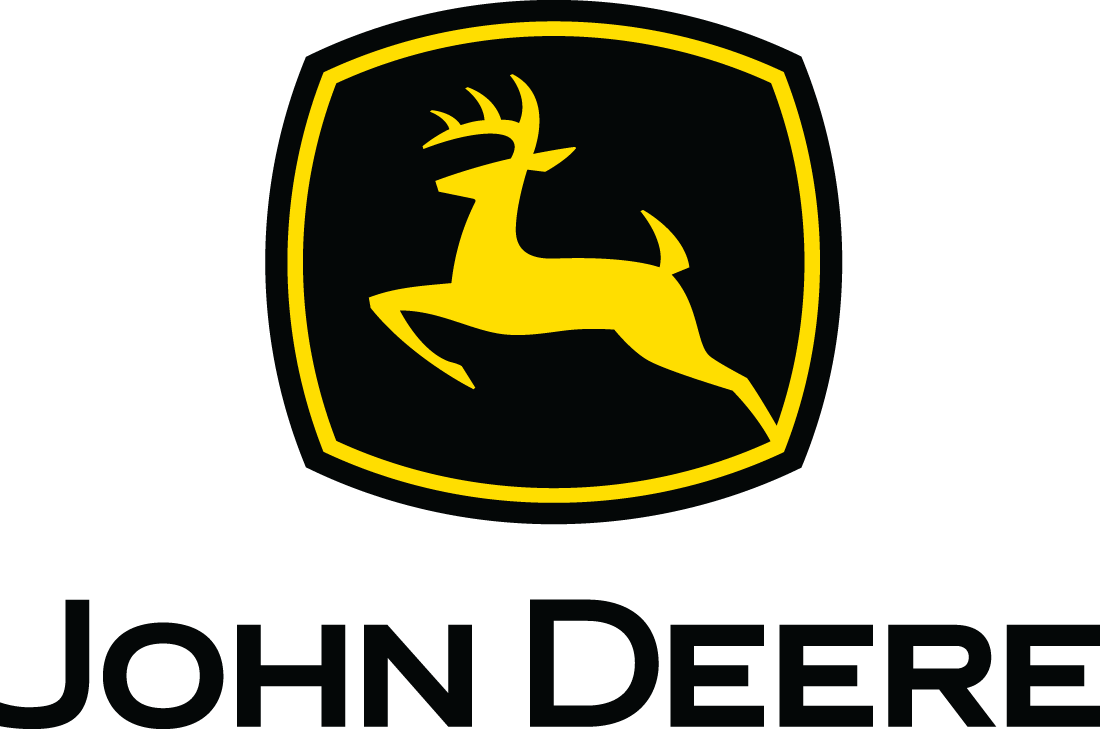
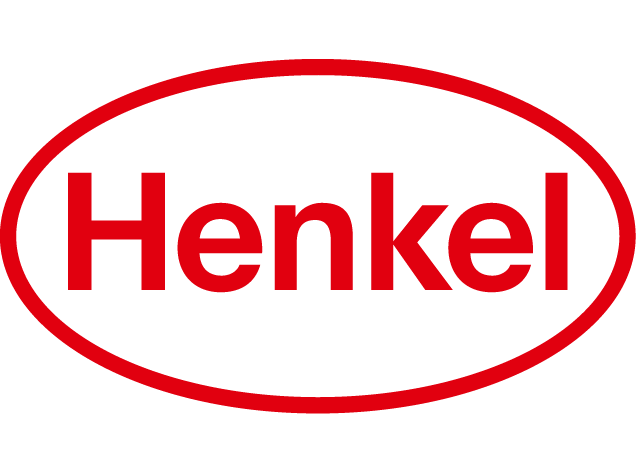
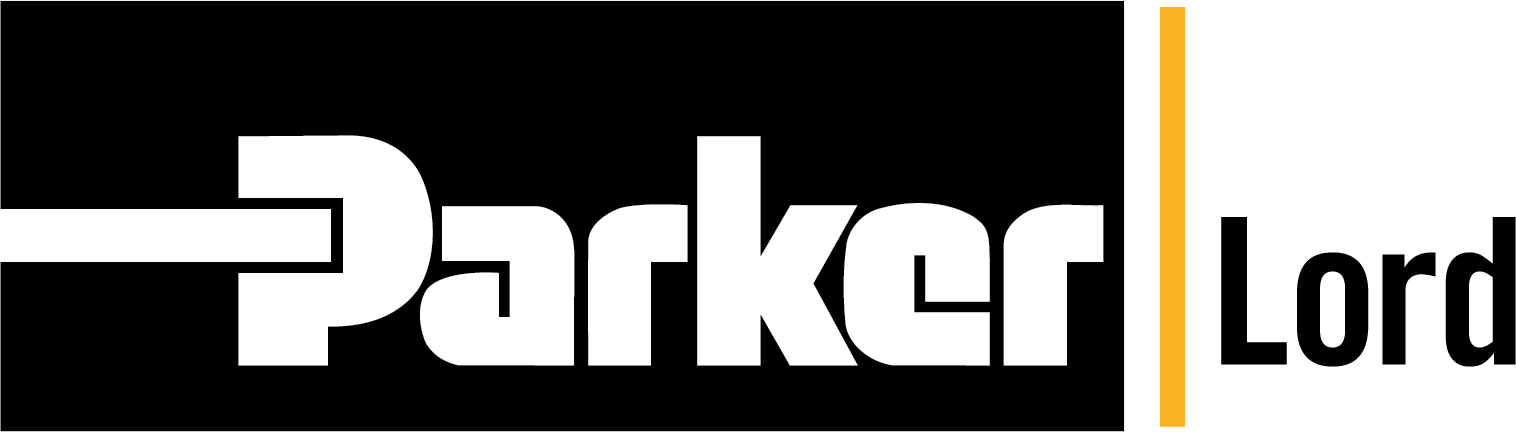
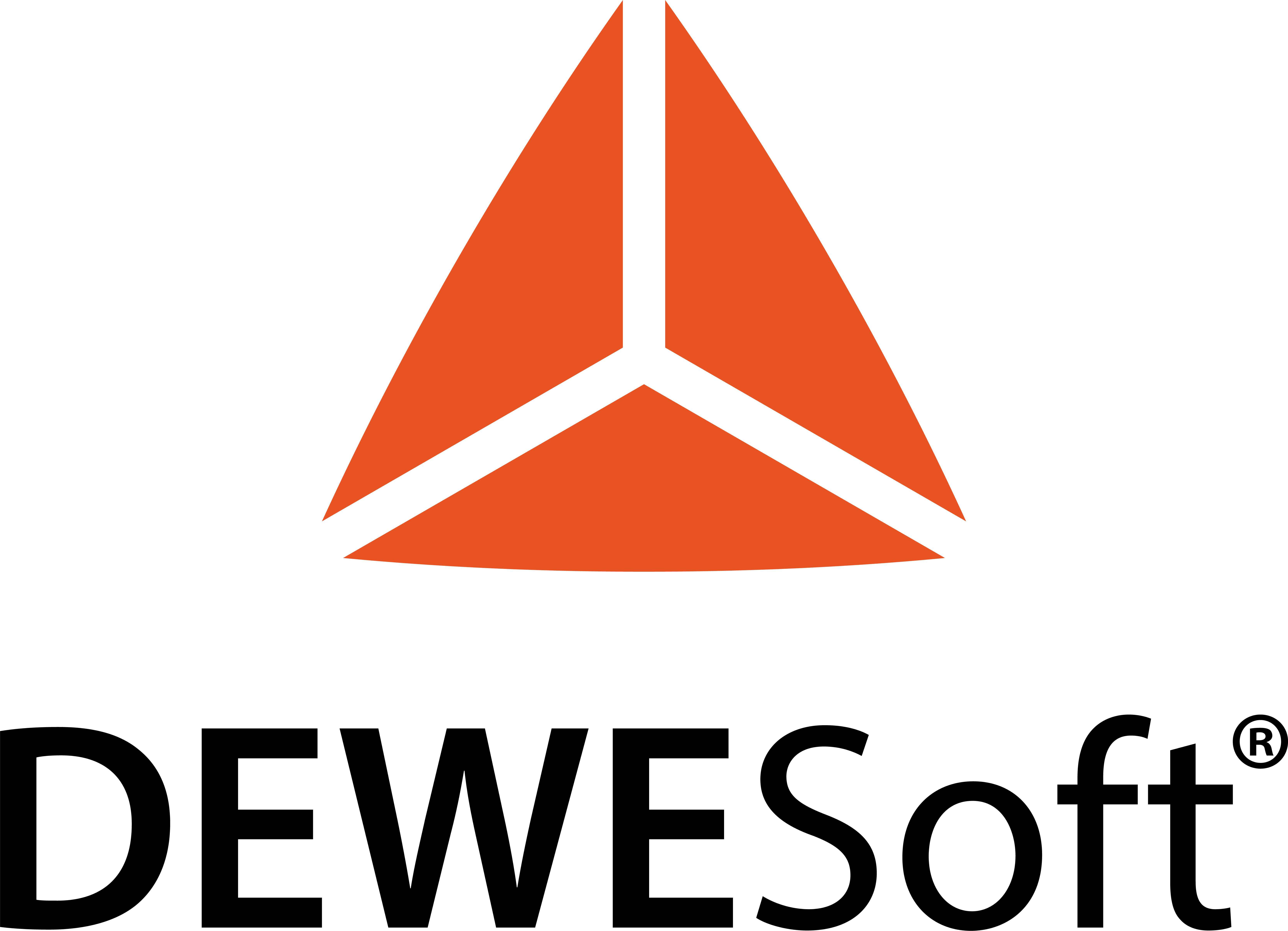
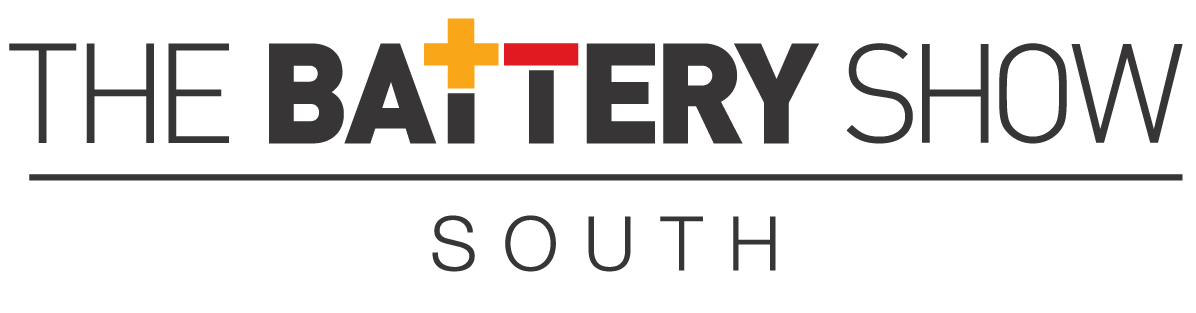
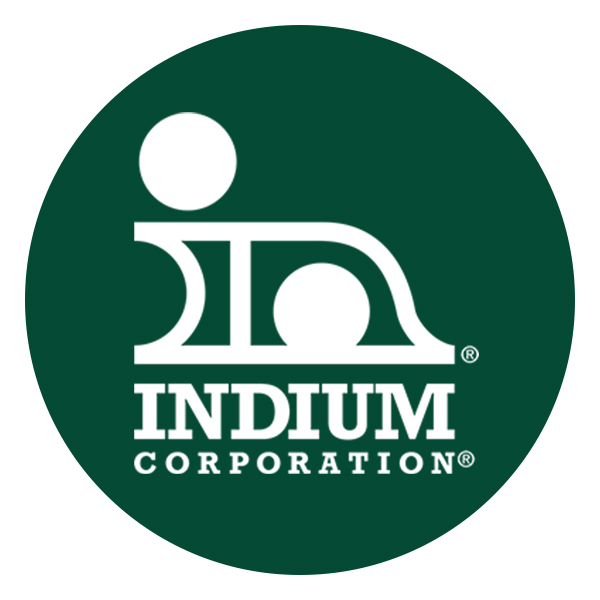
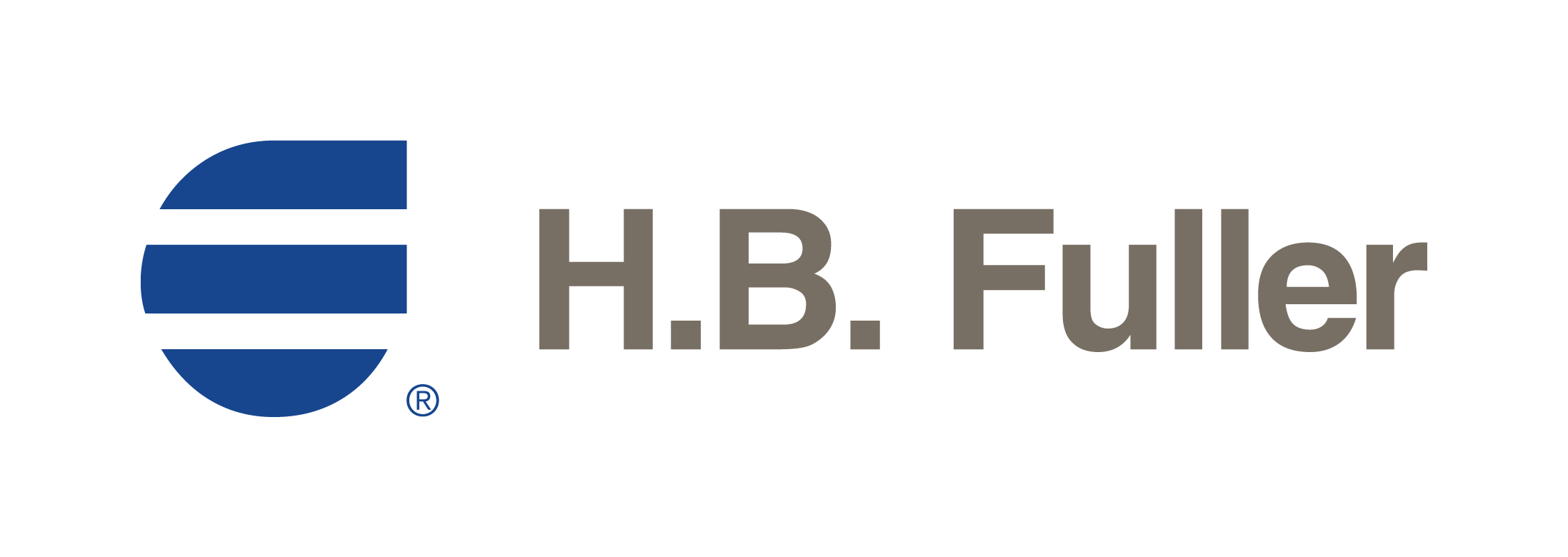