In conversation: Dr Richard Ahlfeld, Ph.D.
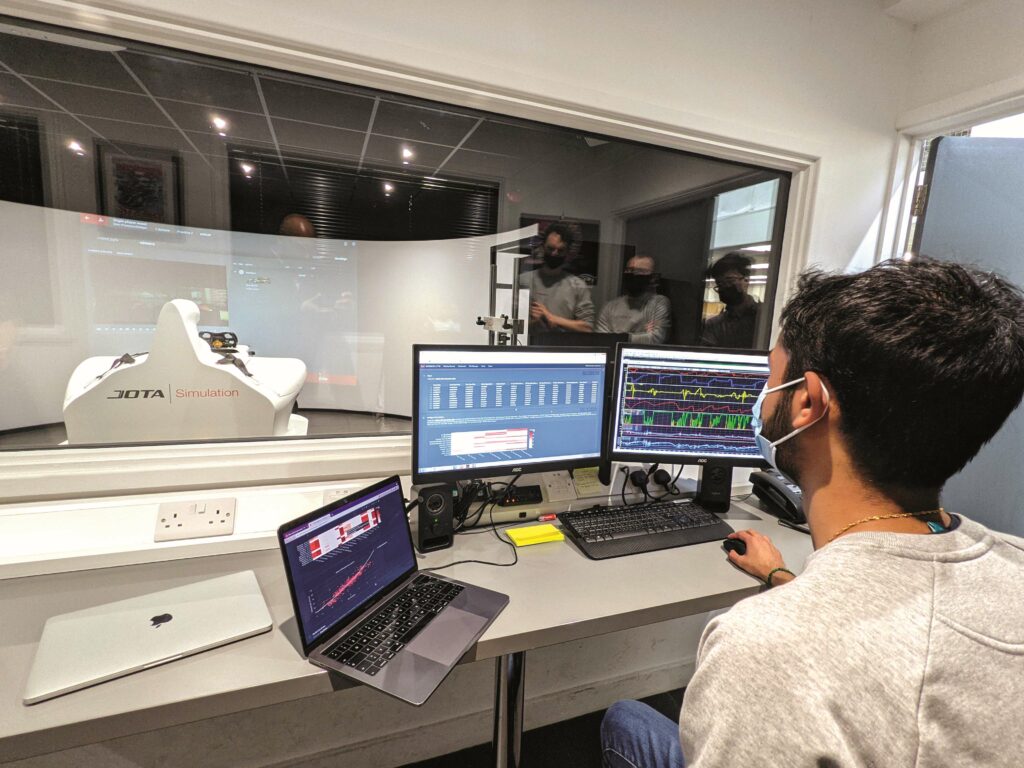
(Images courtesy of Monolith)
Rules of the game
Monolith’s CEO tells Rory Jackson how AI can help improve and shorten vehicle and powertrain development
For much of the 21st century, automotive applications of big data have been limited to some attempts at predictive maintenance in vehicles and powertrains.
This is beginning to change, with the growing use of analytics by many major organisations to characterise current and future battery cell chemistries, based on vast testing data, and to optimise vehicle qualities such as structural integrity, aerodynamics or power efficiency, based on accumulated test data on respective parameters.
However, the benefits of analytics depend on the algorithms at their core. Naturally, a more intelligent algorithm could more accurately predict a cell’s future behaviour, generate a more aerodynamic fairing geometry with a higher success rate, and yield myriad other benefits for battery and vehicle development.
Creating such an algorithm thrust Dr Richard Ahlfeld, chief executive officer of Monolith, into the public spotlight in 2016. At that time, he had been working with NASA to explore how the next Mars mission, the Artemis project, or other space systems could benefit from his work on machine learning for complex engineering tasks.
He presented his research to wide and positive acclaim from NASA’s global supporters, with someone immediately approaching him afterwards to try to license his technology.
“As I was still an employee at Imperial College, I couldn’t license anything, so I went to the university, told them that people wanted to buy my algorithm, and with their encouragement, we founded a company,” Ahlfeld recounts.
Since founding Monolith in 2016 as a spin-out from Imperial, Ahlfeld and the company have worked with not only NASA but also McLaren Automotive, BMW, Honeywell, Mercedes, Michelin, Siemens, among others, to improve and shorten vehicle and powertrain development roadmaps using AI.
“The goal was always to examine how engineers could adapt to this new world in which terabytes of data were being produced by organisations making new aircraft engines, new cars and so on,” Ahlfeld says.
“So, we built our Monolith software platform around this idea of making it easier for engineers to take sensor data, vehicle data and so on, and stream it into a processing engine that figures out key answers to specific questions and problems that they have.”
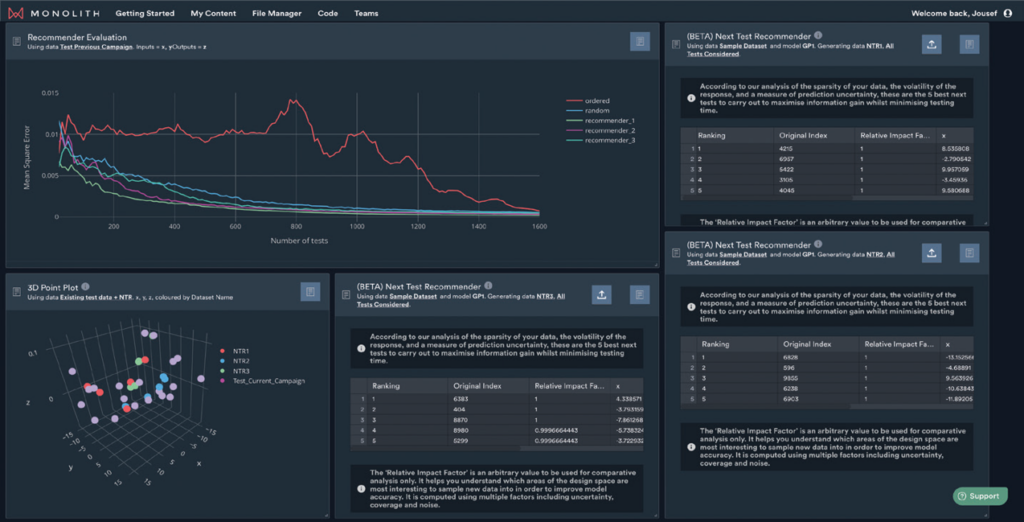
The problem of data
For a tangible understanding of how AI can make a difference to e-mobility, Ahlfeld puts forward the example of battery testing.
“In many cases, the first decision you need to make when designing a new EV is choosing a cell chemistry for your needs, and today we’re on the cusp of sodium-ion, solid-state, aluminium, silicon anode, and other new cell technologies becoming commercially available, but it is most likely you will pick an NMC chemistry today,” he says.
“You need to prototype modules and packs around that, but essentially, no-one really knows how a cell behaves down to its electrochemical elements. It’s incredibly complicated and intractable, and that is the reason some cells die after 12 months and others after 60 months. It is true for current batteries and even more true for next-generation batteries.”
Hence, the only way to believably tell someone if their new EV (and its pack) will last for five years is to take hundreds (sometimes thousands) of the pertinent cell type and cycle them in test machinery, charging and discharging them exhaustively across different environmental conditions. As a result, every major OEM and many other organisations worldwide are investing in cavernous cell-testing labs full of multi-channel battery testers.
The average battery testing lab is a project worth hundreds of millions of dollars, designed around creating masses of data, with each organisation operating their lab independently, without any sharing of best practices or data between companies that could make the work shorter or easier.
“We have tried in the past to get groups to publicise data, especially when it has been scientifically super-relevant, but the reality is: anyone who is investing close on billions of dollars in testing and characterising cells to get them to market is adamantly against giving any kind of advantage or help to their competitors. No-one is sharing information,” Ahlfeld says.
This reticence to share battery testing data, even between labs testing the exact same cell model, leads to a few problems. For one, it means companies must spend (and arguably waste) months of time and effort generating 1-2 TB of cell data per week to understand how long the cells will last in application. As such massive quantities of data are too much for humans to realistically analyse, AI analytics are of obvious use.
Second, battery testing labs will experience more cell failures than those seen in EVs, e-bikes and smartphones, from minor cell swellings to severe leakages and explosions. These tend to occur in controlled spaces without risk to researchers, but can still cause delays and setbacks to module development, lasting potentially months.
“If you’re supposed to release an EV in three years – which China is achieving, though in Europe it still takes maybe five – and you’ve wasted six months testing a cell only for it to go bang and cause you to start from scratch, that is of potentially huge consequence on your time-to-market,” Ahlfeld notes.
Lastly, organisations cannot be certain of the most efficient test plans, encompassing the numbers of cells and cycles per cell, and ranges of conditions to repeat tests under, along with other parameters and permutations.
“This, from my view, is the biggest problem in battery testing: there are so many conditions to test that the default fallback strategy for most OEMs is to test everything, so you end up with more than 800,000 possible test combinations of cell data; each test takes between six and 18 months. They’re wasting huge amounts of money, two to five times what they should be spending, from our experience,” Ahlfeld says.
“But the longer you’ve been in the game, the better you’ve learned how to safety-rate a cell using a shorter and smarter test regimen. Peter Attia, who used to lead battery testing at Tesla, was a Stanford researcher specialising in AI for battery testing. He proved he could reduce the time and cost of battery testing programmes by something like 98%. Within the controlled limits of battery tests, AI can learn how to solve these problems much faster than humans.”
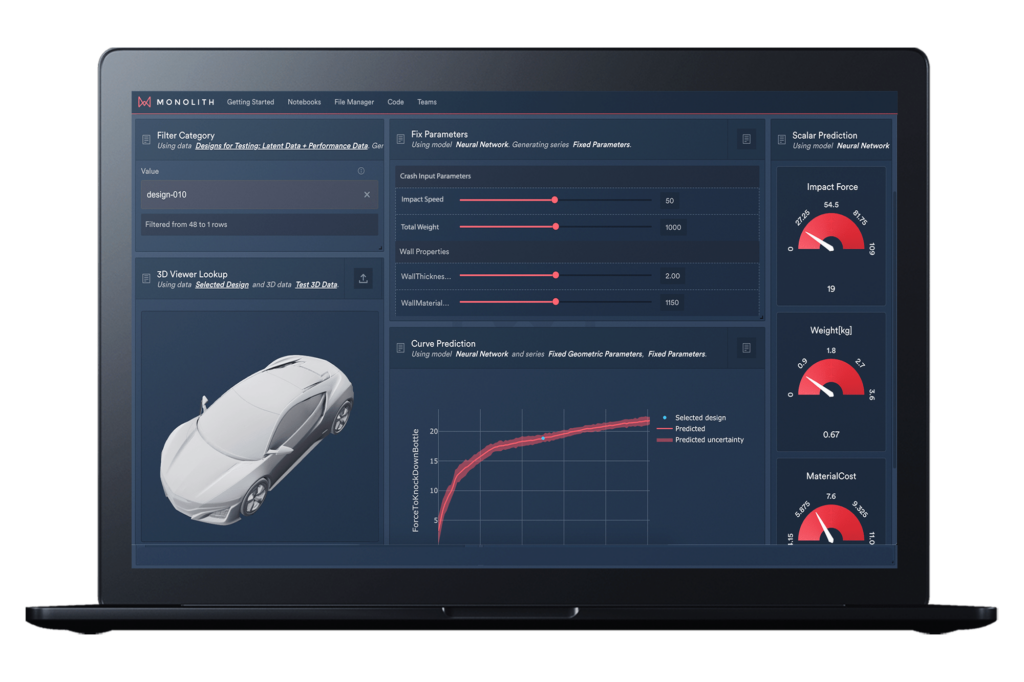
How AI learns
Monolith’s AI platform functions on different types of learning, depending on the application. For optimising a battery test plan, the algorithm goes through a sequence of steps analogous to learning the rules of a game.
“Once it learns the rules, it learns to optimise its next move. It is essentially reinforcement learning, with specific Bayesian optimisations to make it work robustly in dimensions with high noise-to-signal ratios, like battery tests, which often output very jagged, erratic curves in cells’ performance parameters,” Ahlfeld explains.
“Inference is the most important thing: we give the platform a data set, and it has to draw conclusions and assumptions from that set in order to optimise the next one. It is an entire area of machine learning, and we’ve gone through all types available over the last five years, applied them to real test lab environments and picked the ones that worked, adding on user interfaces that make them easier to interact with.
“For our test lab optimisation toolbox, users upload the results they have so far, and the algorithm goes through a search to figure out the rules of the game and the final goal in order to output recommendations.”
He likens the fundamental behaviours of the toolbox to how some social media apps recommend content. From an algorithmic perspective, both types of system must work in noise-heavy environments and infer what should be chosen next, based on past choices (be they clicks, or the number and types of cell tests).
While there are variations, fundamentally, each solution in the Monolith platform has been engineered in the same way: the team has identified an ideal machine-learning tool for solving the specific problem of how to test a battery faster, and then made it easier for engineers to work with it.
AI in battery testing
Three AI solutions within Monolith stand out with regard to battery testing. The first is its Anomaly Detector software, which monitors users’ test stations 24/7, and upon detecting something indicative of a fault condition, alerts them to stop the test.
“In predictive maintenance, you have a finished product with well-established behaviours. With new battery cells, you don’t know how they’re supposed to work, but with this solution’s self-learning, deep learning-based algorithm, just 20 seconds of training it with a ‘golden run’ of ideal test results is enough for it to start learning what is normal for safe cell behaviour, thereafter telling you – almost in a paranoid way – whenever a cell does something unusual,” Ahlfeld says.
The system can work across hundreds of channels with different sensor types, tracking and judging not only voltage or current sensors but also accelerometers, gyroscopes, torque sensors and other automotive-testing equipment.
“Lots of things can trigger an alert to the Anomaly Detector in battery tests. We have seen it flag when a cell swelled up slightly or overheated, when a sensor drifted over time, technicians running the wrong script, unusual vibrations caused by a researcher doing a dance nearby, and it can be configured to prioritise certain signals over others,” Ahlfeld notes.
“Users don’t have to fine-tune an advanced deep neural network. They just label acceptable data signals as okay, so the system learns what is normal behaviour for a cell, and hence what isn’t normal or acceptable, be it overheating, overcurrents and so on.”
The second solution is a Next Test Recommender, which formulates a cell-testing plan (or the remaining parts of an incomplete test plan) based on driving cycles, charging habits, ambient environmental conditions and other factors that the pack will be subjected to.
Naturally, the rate of error in one’s prediction models for cell ageing will be very high when testing starts and drop as tests conclude. If AI is applied correctly to train one’s models, the rate of error drops much more sharply against the rate of tests, so the Next Test Recommender routinely reduces the number of tests that its users need to run by 30-60%.
“That system is very similar to the sort of reinforcement learning that became popularised through Google DeepMind years ago. It basically treats battery testing as a game with specific rules, and essentially the victory condition is discovering with very high certainty how long the battery will last, when it will get too hot and those sorts of end-of-life conditions,” Ahlfeld explains.
“If you’ve looked at Google Go, or any other chess robot, then you know this is something AI has been good at for decades. If you give AI the rules to a game, it can usually learn them much better and faster than a human can, and become very hard to beat. So, it’s very hard to beat an AI at building a test plan if you’re trying to do it yourself manually, and each test costs you time and money, so there is no sense in not using AI to do it.”
The last solution for battery testing is Monolith’s Early Stopping Model, which forecasts the results of the remaining planned tests (typically up to six to 12 months ahead) and aims to judge whether running the remaining tests on the plan is unnecessary; for instance, if a cell seems unfit for purpose, based on test results, then it makes little sense to AI to continue characterising it.
“In many cases the AI can, for instance, see faster than a human when a cell is ageing too quickly, and when it makes no sense to go all the way to, say, 5000 cycles if, after 800 cycles, you’ve got a whole batch of them that are degrading faster than is ideal,” Ahlfeld says.
Future intelligence
Beyond cell characterising, Monolith can be applied to anything with a test prototype to be validated, sensors to extract data from, and at least a moderately complex range of different conditions across which that prototype should be tested (to ensure the system is being used to its full potential).
“For instance, if you are investigating tyre dynamics or friction in automotive or motorsport, you have so many different conditions that you need to run the tyre in that it quickly gets mind-boggling. Similarly, if you’re in some facet of powertrain optimisation or dyno testing – for example, maybe motor durability testing – you have hundreds of different scenarios to deal with,” Ahlfeld says.
“If you have a prototype motor, you need to figure out the best combinations of test parameters, how long you need to test it for, and how far you can trust the data. Empowering engineers to quickly figure that out is what we sought to achieve through Monolith AI since founding the company eight years ago.”
Today, Ahlfeld and his team are shifting their work towards visualising what laboratories will look like five to 10 years into the future, particularly as generative AI makes it possible to produce code or compile test reports faster than ever before.
“Using AI for anything language-based is a lot easier now than it was five years ago, but ChatGPT will never be able to understand the intractable physics of batteries,” he says. “If you could ask ChatGPT how to make a functioning sodium-ion battery, that would be great, but it can only learn off the internet, and no-one on the internet has solved that yet.
“Advanced thermal-management techniques, new battery chemistries, tyre cooling and plenty of other vehicular problems will always require a real, physical laboratory for experimenting and investigating to see what works.
“But if we can build the AI algorithms behind those labs to allow, say, faster discovery of new battery materials, next-generation powertrain configurations and so on, we can bring the future, high-throughput test laboratory to life.”
Dr Richard Ahlfeld
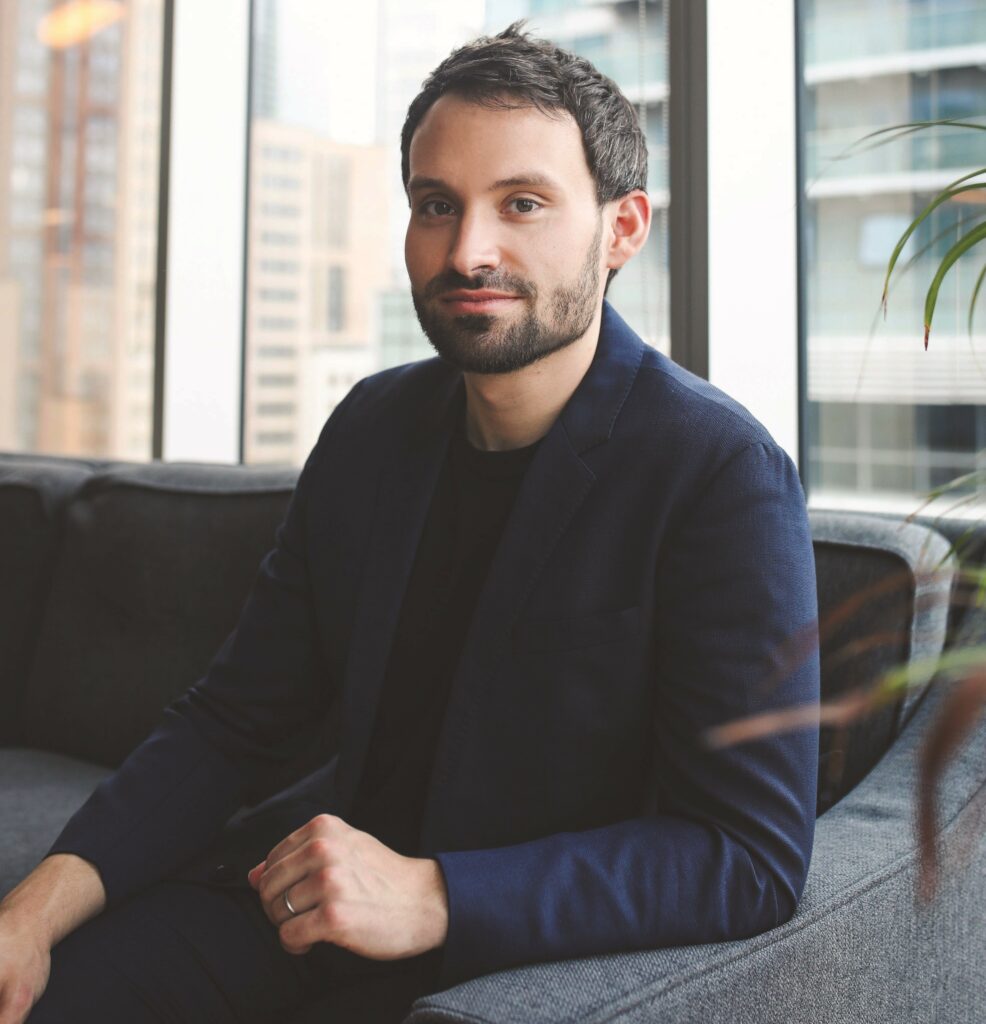
Dr Richard Ahlfeld was born in Hausen ob Verena, near Munich, Germany, and he achieved his Bachelor of Engineering degree at Bundeswehr University in 2010 (in Mathematical Engineering).
He went on to study Aerospace Engineering and Mathematics in a Master’s capacity at Delft University of Technology from 2011, briefly working as an intern at MTU Aero Engines that year, before graduating Summa cum Laude and with Honours in 2013 (completing his Master Thesis at Airbus Defence and Space).
After completing a PhD in Aerospace Engineering and Data Science in 2017, at Imperial College London, he founded Monolith AI. Soon after, the company started its first paid work with McLaren Automotive, aimed at reducing a new supercar’s early-stage engineering deviations and accelerating its virtual validation lifecycle.
Today, he continues to lead Monolith as CEO. In his spare time, he plays the piano, and he has written articles for The AI Journal as an editorial contributor since 2021.
Click here to read the latest issue of E-Mobility Engineering.
ONLINE PARTNERS
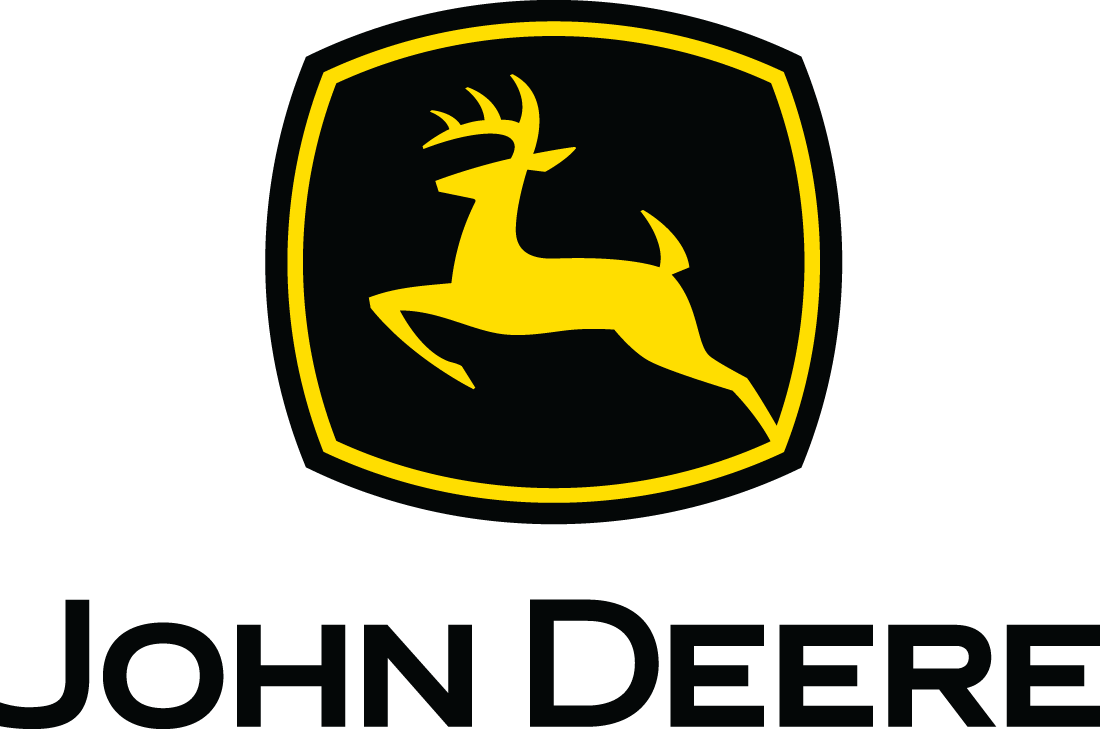
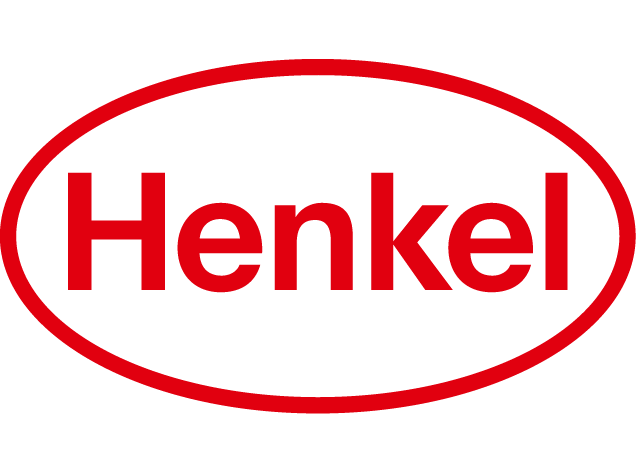
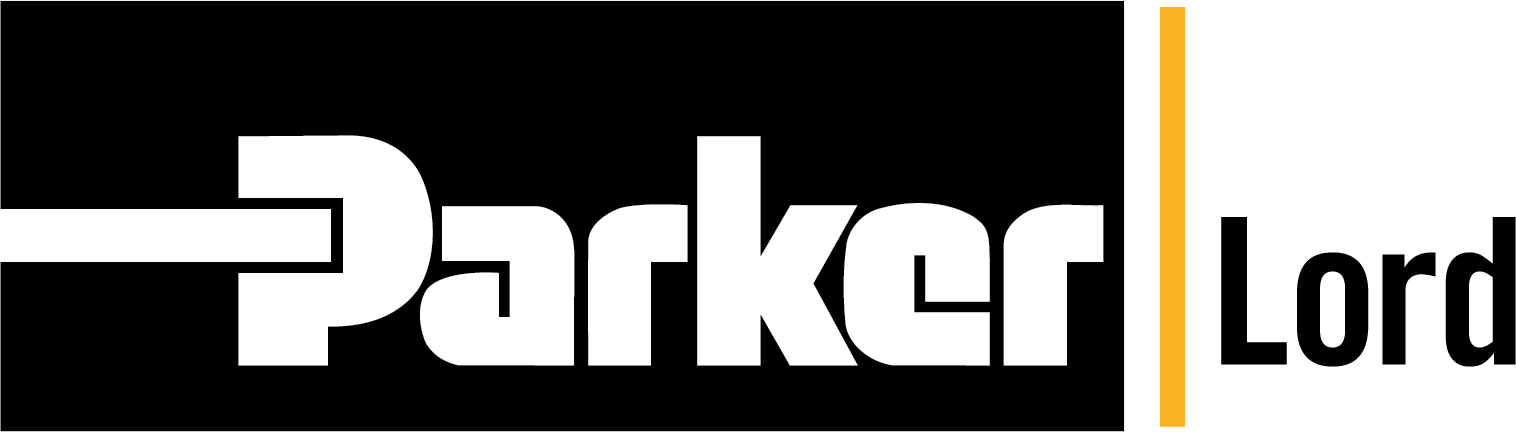
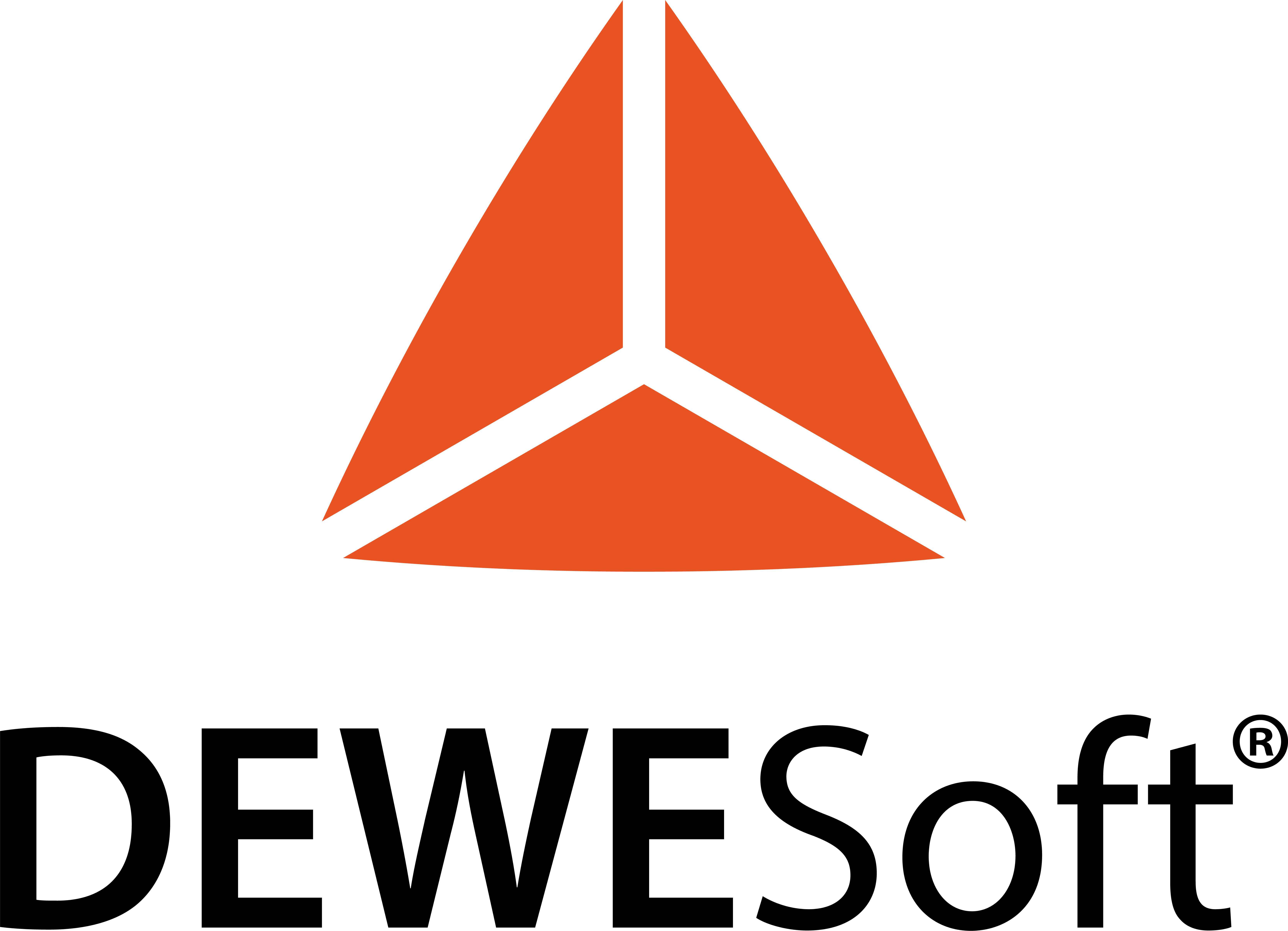
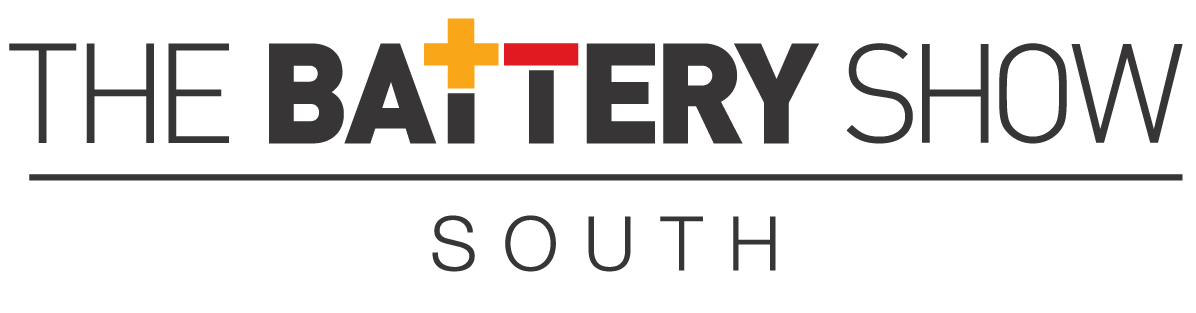
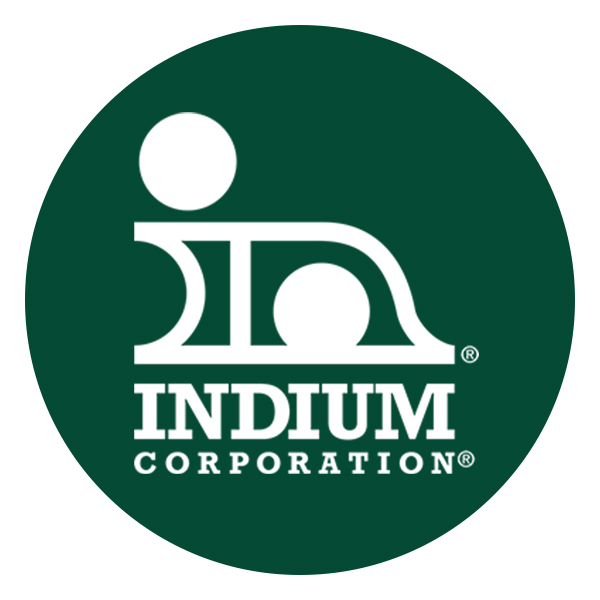
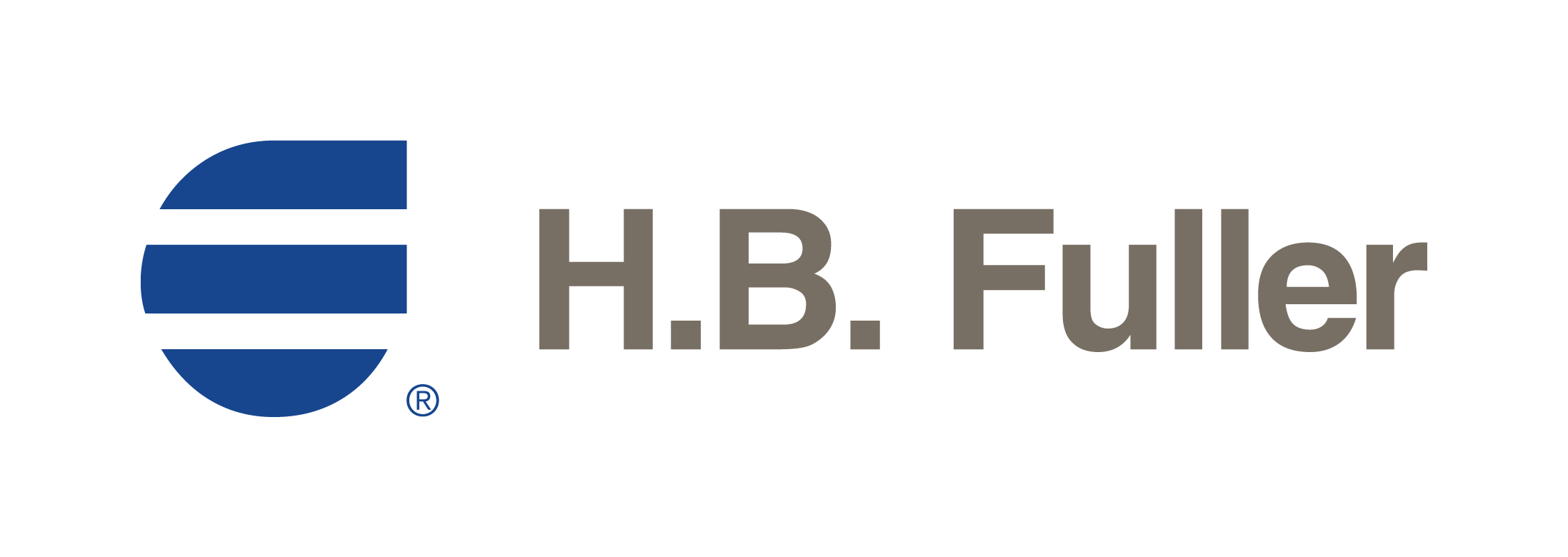